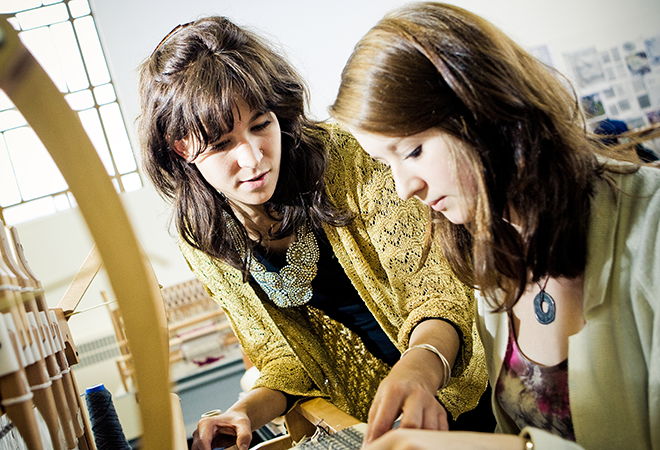
Predictive Working Tool for Early Identification of ‘At Risk’ Students
Status
Completed: 21 August 2010
Project Details
A project completed in 2010, undertaken by Open Polytechnic, to develop a predictive working tool of student persistence based on methods of multivariate statistical analysis that is general enough and applicable in any other contact or distance education institution in New Zealand.
Aims:
The main aims of the project were to:
- explore the variables that may influence persistence or dropout of students at the Open Polytechnic
- determine whether the differences in student background characteristics could be used to distinguish between the likely retention rates of persistent and non-persistent students
- determine the extent to which data captured by the enrolment form could help us to identify future successful and unsuccessful students before the course began.
Methodology:
The project methodology involved:
- quantitative data analysis of students’ enrolment records using statistical software
- descriptive and exploratory data analysis to summarise the data
- multiple and logistic regression, classification and regression trees and discriminant analysis to identify relationships between variables, to quantify the impact that factors have on persistence, and factors which contribute most, and to discriminate between groups of students.
Team
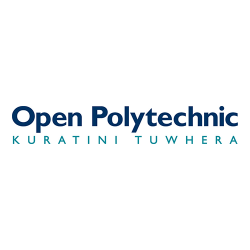
Associate Professor Zlatko Kovačić
Project Leader
Open Polytechnic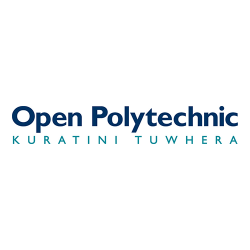
John Green
Open PolytechnicStatus
Funding
$10,000.00 (excl GST)
Key Findings
The key findings from the project included:
- The empirical results show that the most important factors separating successful from unsuccessful students in order of importance, were: ethnicity, course level, secondary school qualification (highest level of achievement held from a secondary school), programme and age.
- Ethnicity is not something a student can change or an institution can influence, but advice on the most appropriate study options for that student, i.e. distance, online or contact study may be provided. Would some students be better served by studying in a contact institution, or if in a contact institution, by distance?
- The factor course level is deceiving, as it might suggest that students studying a lower level course are more likely to succeed. In fact, the reverse is true. Students on higher level courses who have already proven themselves in lower level courses are more likely to succeed, making this a relatively predictable result in much the same space as the third factor, secondary school qualification. Previous academic success is a strong indicator of future academic success and has been used in the UK by University Matriculation Boards for decades.
- Advising a student that a different degree programme might increase their chances of academic success appears to be fraught with difficulties but in a larger institution with more choices of programme it may be quite appropriate.
- Like ethnicity, age is not something a student or institution can modify but it should come as no surprise that younger less mature people have less motivation than older more mature people who generally have a higher motivation to succeed.
Key Recommendations
The key recommendations from the project included:
Student support systems | Our results have some interesting practical implications for both academic and administrative staff at the Open Polytechnic. Classifying students based on pre-enrolment information and the rules presented for each node in the classification tree would allow the administrative and academic staff to identify students who would be ‘at risk’ of dropping the course even before they start with their study. Then the student support systems, such as orientation, advising, and mentoring programmes or tertiary study skills courses or compulsory pre-tests, could be used to positively impact the academic successes of such students.
Implications for academic and administrative staff | The implications of these results for academic and administrative staff are several. The implications of identifying a student as potentially unsuccessful must be considered. If the student is told how they have been categorised what effect might this have on their self-esteem and subsequent motivation? In tough economic times should the organisation refuse to enrol students statistically unlikely to pass the course? Or should they allocate further resources to support those students with no guarantee that this support will be effective?
Classification methods | Classification using discriminant functions was the most accurate overall but required the consideration of more factors and was less accurate in identifying ‘at risk’ students. The CART classification tree was the most accurate. Regardless of the method used, our results suggest that using enrolment data alone is only moderately successful in separating successful from unsuccessful students.
Results are specific to the population analysed | It is essential to recognise that while this model will effectively separate successful and unsuccessful students with a good level of accuracy the results are specific to the population analysed. The results would need to be regularly updated with each passing trimester and for a different student population. This would allow each unique student body to be modelled and a checklist used to identify potentially unsuccessful students prior to enrolment.
A research report prepared by Zlatko Kovačić and John Green.
(PDF, 2.42 MB, 73-pages).
- 20 August 2010